We live in a world full of mechanical components and machines that we use day by day. All machines run to failure at some point. An unexpected breakdown of domestic appliances affects your mood or your monthly budget, while any failure in manufacturing can cost millions of dollars.
Every company needs to control the quality of equipment and organize regular maintenance. It can reduce the probability of failures and possible losses for a company. Among the strategies to preempt these failures, adopting predictive maintenance solutions and harnessing machine learning for predictive maintenance stand out as pivotal. Just as machine learning is crucial in predictive maintenance, it's also transforming other sectors, such as finance. To understand its impact in another domain, you can read the article on AI credit scoring.
This article may be relevant to Data Analysts and specialists within the Machinery industry.
What is Predictive Maintenance? An Overview of Predictive Maintenance Techniques
Predictive maintenance is a technique of continuous monitoring of equipment performance and defining its condition. Such monitoring during normal operations helps to detect possible defects and successfully predict failure in advance. The main goal of predictive maintenance is to reduce unplanned glitches or downtime of equipment.
Predictive maintenance makes it possible to conduct timely technical support. This approach involves periodic inspections and repairs of defects with the goal of avoiding equipment malfunction or failure.
As for any data analytics, you will need historical data and ML algorithms to organize predictive maintenance. The success of predictive maintenance models depends on three main components: having the right data available, framing the problem appropriately, and evaluating the predictions properly. This process is similar to the advanced fraud detection methods used in machine learning, as detailed in advanced fraud detection using machine learning, where historical data and ML algorithms play a crucial role in identifying and preventing fraudulent activities. Additionally, the importance of accurate data and proper problem framing can be seen in AI-based credit scoring, as explored in "credit scoring using machine learning", where these factors are vital for assessing credit risk accurately.
Main Models and Approaches Used for Predictive Maintenance
Predictive maintenance can be done by utilizing the process of anomaly detection. Algorithms show you any abnormal behavior of the equipment, thus, you will be able to detect and resolve any encountered issues. As well as monitoring the ongoing processes, you can identify if there's a possibility of future failures.
Here, a comprehensive understanding of equipment behavior metrics is pivotal. As a result, companies can effectively forecast and prototype their equipment's future condition through predictive maintenance technologies
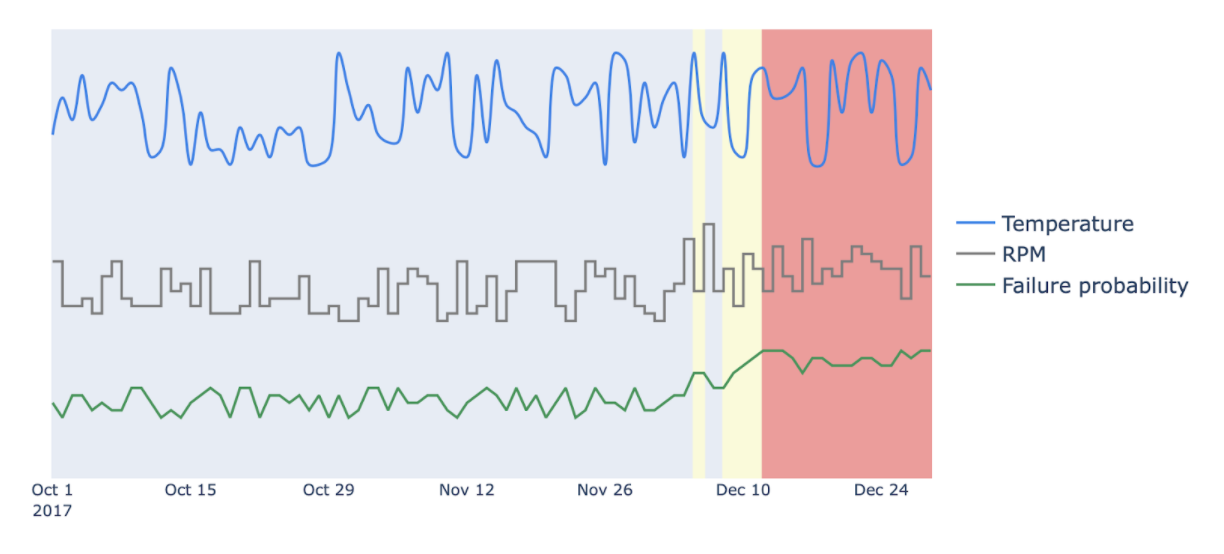
Regression models to predict remaining useful lifetime (RUL)
The Remaining useful lifetime (RUL) is the length of time equipment is predicted to operate before it requires repair or replacement. Regression models for the RUL require available static and historical data with labeled events.
The data that can be used to determine RUL is the following:
- Data that indicates how long similar equipment worked before it reached failure
- Run-to-failure data collected from similar machines
- A known threshold value of a condition indicator that detects failures
As a result, you will have information about how many days or cycles are left before the system fails.
Classification models to predict failure within a given period
If you decide to create a model that can predict lifetimes very accurately, it can be very challenging and time-consuming. However, usually, you don't need to be very precise when it comes to failure prediction. The information that "the equipment is going to fail soon" often is enough. As with the regression models for RUL, you will need both static and historical data with all events labeled. In fact, regression and classification models are pretty similar, but mostly differs on:
- Degradation process. Since we are defining failure in a time window instead of an exact time, the requirement of its smoothness can be relaxed
- Classification models can deal with multiple types of failure, as long as they are framed as a multi-class problem
- There are "enough" cases of each type of failure to train the model
As a result of such analysis, you will know if a machine fails in the next period of time.
Detecting anomalous behavior approach
In some cases, analysis of the failures doesn't work. In lots of different industries, the is very little information about failures or no examples at all. For example, you can't afford to collect data about airplanes or railways crashes, as it's a matter of not only money but potentially human lives. For such cases, you need to monitor the behavior of the vehicle or a machine to figure out if the shown behavior is normal or not.
For such analysis, you will need static and historical data, but there may be labels that are unknown or too few failure events were observed or there are too many types of failure.
It is possible to define what normal behavior is and the difference between current and "normal" behavior is related to degradation leading to failure.
The anomaly detection model is very controversial because of its generality. The model should be able to detect all kinds of failure, even in cases with no previous information about them. At the same time, abnormal behavior doesn't necessarily fail. In cases, where it can lead to failure, the model doesn't provide the information about possible time frames when it might occur.
The analysis of an anomaly detection model is also challenging because of the lack of labeled data. When no labeled data is available, the model is usually made accessible and domain experts provide feedback on the quality of its anomaly flagging ability.
Survival models for the prediction of failure probability over time
A survival model evaluates the probability of failure for a specific type of equipment with static features, as well as it shows the impact of certain features on a lifetime. It provides, therefore, estimation for a type of machine with similar characteristics.
This strategy gives an analysis of how the risk of failure can change in time with the set characteristics.
How to Build Effective Predictive Maintenance Models?
Creating effective predictive maintenance models involves understanding the data, selecting the right algorithms, and continuously refining the model based on feedback and performance.
Here's a step-by-step guide:
Gathering and Preparing the Data
The foundation of any predictive maintenance model is high-quality data. Collecting detailed operational and maintenance data is crucial. This includes historical data on machine performance, maintenance records, sensor readings, and any instances of failures or repairs. Data cleaning and preprocessing are essential steps to remove outliers, handle missing values, and ensure the data is in a usable format for model training.
Selecting the Right Machine Learning Algorithms
Choosing the appropriate machine learning algorithms is key to developing effective predictive maintenance models. Regression models, classification algorithms, and anomaly detection techniques are commonly used, depending on the specific objectives of the predictive maintenance system. For instance, regression models might be used to predict the remaining useful life (RUL) of a machine, while classification models could identify whether a machine is likely to fail within a certain time frame.
Feature Engineering and Selection
Feature engineering involves creating new features from the existing data to improve model performance. This might include aggregating sensor data over time, calculating statistical metrics, or incorporating domain-specific knowledge to highlight relevant patterns. Selecting the most informative features is critical to building a robust model.
Model Training and Validation
With the data prepared and features selected, the next step is to train the predictive maintenance model. This involves splitting the data into training and test sets, using the training data to fit the model, and then validating its performance on the test set. Performance metrics such as accuracy, precision, recall, and F1 score can help evaluate the model's effectiveness in predicting equipment failures.
Implementation and Continuous Improvement
Deploying the model into a production environment is the final step. This involves integrating the model with existing maintenance systems and workflows. Continuous monitoring of the model's performance is essential, as is regular updating with new data to ensure its predictions remain accurate over time. Feedback loops where maintenance teams provide insights on prediction accuracy can help refine and improve the model.
Leveraging Advanced Technologies
Incorporating advanced technologies like deep learning and neural networks can further enhance predictive maintenance models, especially when dealing with complex patterns or large datasets. These techniques can automatically learn and improve from experience without being explicitly programmed, offering potential improvements in prediction accuracy.
Challenges of Predictive Maintenance Technologies and Solutions
While predictive maintenance machine learning methods and predictive maintenance software are revolutionizing the manufacturing sector, they come with their own set of challenges. It's essential for businesses to recognize these potential hurdles to fully harness the power of predictive maintenance.
Here are some of the key challenges to consider:
Data Volume and Quality:
The success of predictive maintenance heavily relies on the quality and volume of data. Ensuring that data is accurate, relevant, and consistently available can pose a challenge.
Defining "Normal" Behavior:
With myriad predictive maintenance tools available, determining the standard or "normal" behavior of equipment can be daunting, especially with evolving machine standards and practices.
Complex Implementation:
The line between predictive maintenance vs preventive maintenance can sometimes blur. Determining which approach to prioritize and integrating them harmoniously can prove complex.
High Initial Costs:
Investment in cutting-edge predictive maintenance technologies and software solutions can be substantial. While the returns are significant in the long run, the initial outlay can deter some businesses.
Training and Skill Set:
Understanding and leveraging predictive maintenance solutions requires a specialized skill set. Training personnel and ensuring they are up-to-date with the latest methodologies can be a continuous challenge.
Interpreting Predictive Data:
While predictive maintenance machine learning models can provide a wealth of data, interpreting this data correctly and making informed decisions based on it is crucial. Misinterpretations can lead to erroneous actions, defeating the purpose of predictive maintenance.
In conclusion, while the predictive maintenance definition underscores its vast benefits, addressing these challenges head-on is crucial for businesses to maximize their potential.
Key Advantages of Predictive Maintenance Software and Technologies
Predictive maintenance is elevating manufacturers to new operational heights. By blending advanced technology with deep learning insights it not only optimizes machinery function but also fortifies long-term business investments. Let's explore its multifaceted benefits:
- Cost Efficiency: Predictive maintenance considerably diminishes the expenses related to both routine screenings and unforeseen equipment failures.
- Prolonged Equipment Lifetime: Proper predictive maintenance solutions can substantially extend the operational lifespan of machinery and equipment.
- Enhanced Safety and Service Quality: Implementing predictive maintenance machine learning models minimizes risks, ensuring heightened safety for users and improved service quality.
- Significant Financial Impact: When executed correctly, the financial returns from predictive maintenance vs. preventive maintenance can soar into millions, contingent on business scale and application specifics.
- Machine Learning Advantages: Utilizing machine learning for predictive maintenance brings forth a more dependable, cost-effective strategy. It utilizes time-series data, usually sourced from sensors, to fine-tune maintenance schedules.
- Minimized Downtime: One of the standout benefits of predictive maintenance is the substantial reduction in unscheduled equipment downtimes, leading to immense cost savings.
- Trust and Transparency with Customers: A well-implemented predictive maintenance strategy fosters trust, ensuring transparent interactions with clients.
Predictive vs. Preventive Maintenance: Key Differences
1. Basis of Action:
Predictive Maintenance (PdM): Operates based on real-time equipment conditions using data-driven insights. It waits for a specific set of criteria to be met before action is taken.
Preventive Maintenance (PM): Follows a pre-determined schedule, either based on time intervals or equipment usage.
2. Cost Efficiency:
PdM: Can be more cost-effective in the long run as it targets specific issues, minimizing wastage of resources.
PM: This can sometimes lead to over-maintenance, resulting in potentially unnecessary costs.
3. Risk of Equipment Failure:
PdM: Reduces the risk of unexpected failures by constantly monitoring equipment health.
PM: Mitigates the risk based on historical data, but there's still a possibility of unpredicted failures.
4. Implementation Complexity:
PdM: Requires sophisticated tools, sensors, and data analytics platforms for accurate predictions.
PM: Typically simpler to implement, relying on schedules derived from manufacturer recommendations or past experiences.
5. Flexibility:
PdM: Offers more flexibility, adjusting maintenance activities based on actual equipment conditions.
PM: Has less flexibility due to its fixed maintenance schedule.
In essence, while preventive maintenance sticks to a set routine, predictive maintenance adjusts its approach based on real-time data analytics, ensuring machinery operates optimally for longer periods.
Use Cases of Predictive Maintenance with Machine Learning
How does Tesla use predictive maintenance
One of the greatest examples of using predictive maintenance is Tesla. Their cars are known as one of the most innovative and reliable, and they keep improving their products. Tesla has recently implemented a new initiative: Tesla cars can now self-diagnose internal problems and order replacement parts from the diagnostic center. A car monitors conditions of all the elements, analyses it, and shows a notification of an issue with the car's power conversion system. The car automatically places an order for replacement parts to be delivered to a Tesla service center, where the owner's car would be repaired.
Predictive Maintenance in the Aerospace Industry: Boeing's AnalytX
Boeing, one of the largest aerospace companies in the world, leverages predictive maintenance to enhance the reliability and efficiency of its aircraft. Through its AnalytX platform, Boeing utilizes vast amounts of data from aircraft sensors and operations to predict potential failures and maintenance needs before they occur.
The AnalytX platform analyzes data from flight operations, maintenance history, and real-time sensor data to identify patterns and anomalies indicative of potential issues. This predictive analysis allows for the scheduling of maintenance only when needed, rather than following a strict time-based schedule. As a result, airlines can reduce unplanned downtime, optimize maintenance operations, and significantly cut costs associated with over-maintenance and aircraft on-ground time.
One notable application of Boeing's predictive maintenance is in monitoring the health of aircraft components like the Auxiliary Power Unit (APU), engines, and hydraulic systems. By predicting when these components are likely to fail or require maintenance, Boeing helps airlines ensure the safety, reliability, and availability of their fleets.
This approach not only enhances operational efficiency but also improves safety by ensuring that potential issues are addressed before they can lead to in-flight problems. Boeing's use of machine learning for predictive maintenance exemplifies how advanced analytics and real-time data can revolutionize maintenance strategies in the high-stakes aerospace industry.
How to integrate predictive maintenance with AI and machine learning
Implementing a machine learning (ML) strategy for predictive maintenance can significantly enhance operational efficiency but involves several complex steps. Initially, it's essential for companies to gather comprehensive static and historical data about their operations and equipment monitoring. Developing an effective ML model from scratch demands not only a substantial dataset but also considerable expertise in data processing, feature engineering, and pattern recognition to accurately predict equipment failures.
To successfully integrate predictive maintenance with AI and machine learning, businesses typically have two options:
- Engage an Expert: Hiring an external consultant or a data scientist with specialized experience in machine learning can provide the necessary expertise to navigate the complexities of predictive maintenance models.
- Adopt Specialized Software: Utilizing advanced predictive maintenance software can simplify the process. These platforms are designed to handle the intricacies of data analysis and model development, making predictive maintenance more accessible.
Finding the right tools and expertise can be challenging, but the investment in AI and machine learning for predictive maintenance can lead to significant operational improvements, cost savings, and enhanced equipment reliability. By choosing the right approach, companies can effectively predict and prevent equipment failures, minimizing downtime and maximizing productivity.
Ready to transform your predictive maintenance with Datrics.ai's ML
Discover how the Datrics.ai platform can empower your team to analyze vast datasets and optimize equipment maintenance with unparalleled ease and accuracy. No deep technical knowledge? No problem. Datrics.ai is designed to simplify complex data analysis, making predictive maintenance accessible to everyone.
Take the first step towards predictive maintenance excellence today. Visit www.datrics.ai to learn more and start your journey to smarter, data-driven maintenance decisions.
FAQs
What is predictive machine maintenance?
Predictive machine maintenance is a proactive approach that uses data analysis tools and techniques to detect anomalies and predict equipment failures before they occur. This method allows for timely maintenance actions, reducing downtime and extending the lifespan of machinery.
How is machine learning used in predictive maintenance?
Machine learning in predictive maintenance involves analyzing historical and real-time data from machines to identify patterns that precede failures. By training models on this data, machine learning can forecast potential issues, enabling preemptive maintenance actions to avoid costly breakdowns.
Which algorithm is best for predictive maintenance?
The best algorithm for predictive maintenance depends on the specific application and the nature of the data. Commonly used algorithms include regression models for predicting time until failure, classification models for identifying if and when a machine might fail, and anomaly detection algorithms for spotting unusual patterns that could indicate impending issues. The choice of algorithm should be tailored to the maintenance goals and data characteristics of each unique situation.